Rather listen than read? Check out this article’s podcast below.
While we often highlight the impressive capabilities of AI, especially LLMs, we also focus on their shortcomings—particularly their tendency to “hallucinate” or generate information that isn’t grounded in reality. But what if these so-called flaws aren’t just quirks of machine learning? What if they reflect fundamental aspects of human cognition? Perhaps we’re more like AI than we’d like to admit.
Intuition and gut feelings
At the core of LLMs is the ability to predict the next word in a sequence based on patterns learned from vast amounts of data. Surprisingly, human brains operate in a remarkably similar way. Our minds are constantly making predictions about the world, filling in gaps in our perceptions and understanding to create a coherent narrative of reality.
Much like LLMs generate responses based on learned patterns, humans often do the same. Humans act because of neurons firing in their brain. Neurons in their brain which are either transferred genetically or shaped by the vast amount of sensory input they received from baby on upward. In practice we call these intuitions or “gut feelings”, and just as LLMs we often make decisions based on them without complete information. This can lead us to conclusions that feel right but aren’t necessarily supported by facts. For example, someone might have a strong opinion on a complex topic they’ve only heard about in passing, filling in gaps with assumptions shaped by personal biases and limited experiences.
False memories and confabulation
Psychological research has shown that we are indeed prone to “hallucination”-like errors. The human memory is highly malleable. Psychologist Elizabeth Loftus has extensively studied the phenomenon of false memories, demonstrating how people can vividly recall events that never occurred. In one study, participants were convinced they had been lost in a shopping mall as children—a completely fabricated event. This process, known as confabulation, mirrors how LLMs can produce detailed but fabricated responses when generating text.
Our brains have a tendency to “fill in” missing information to create a complete picture, which is also how AI Diffusion models work to generate images. The concept of boundary extension in cognitive psychology illustrates that this is indeed happening to humans. When individuals are shown a close-up image and later asked to recall it, they often remember seeing a wider, more expansive view than what was actually presented. This inclination to expand beyond the given information is akin to how Diffusion models or LLMs might generate additional context or details that weren’t part of the original input.
My Warcraft III experience
Many of us have experienced moments where our memories don’t align with reality. Recalling my early days playing the video game Warcraft III, I remembered it having a wide, expansive viewpoint and detailed landscapes. Years later, driven by nostalgia, I revisited the game and was surprised to find the actual view much narrower than I had remembered. This discrepancy highlights how our minds can fill in gaps, enhancing past experiences in ways that align with our perceptions and emotions.
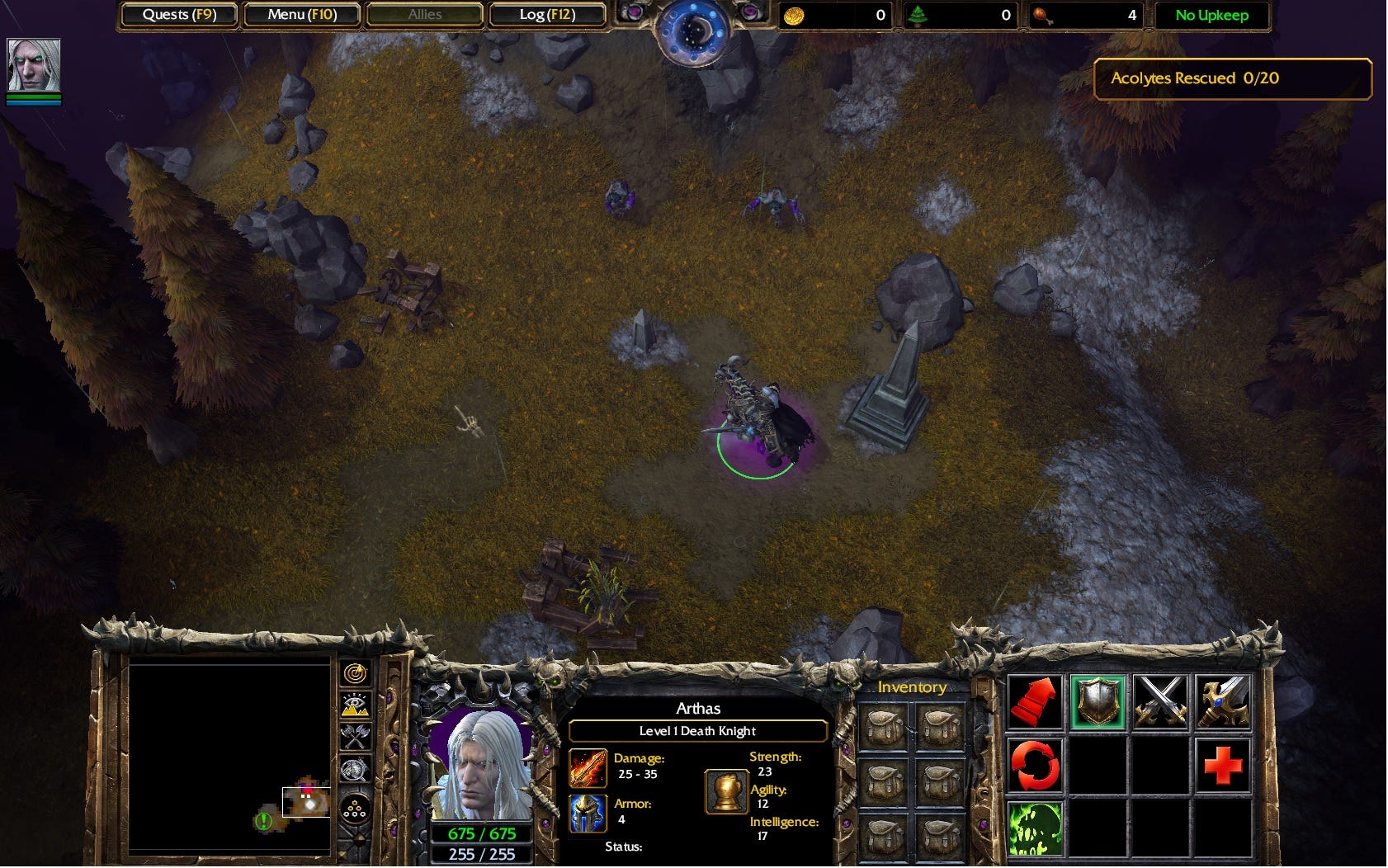
LLMs and the human mind: Filling in the gaps
When faced with incomplete information, both humans and LLMs strive to create coherence, sometimes at the expense of accuracy.
LLMs generate responses by predicting the most probable next word or phrase based on patterns they’ve learned. When asked about unfamiliar topics, they may produce plausible but incorrect information—effectively “hallucinating” facts that fit the context. This isn’t a malfunction but a fundamental aspect of how they process and generate language.
Similarly, humans often make predictions or assumptions to fill in missing information. This can lead to misconceptions or the spread of misinformation (hallucinations?), especially when individuals rely on intuition over verified facts.
Pareidolia: Seeing patterns where none exist
The parallels between human cognition and LLM operations suggest that the “flaws” we identify in AI are, in fact, reflections of our own mental processes.
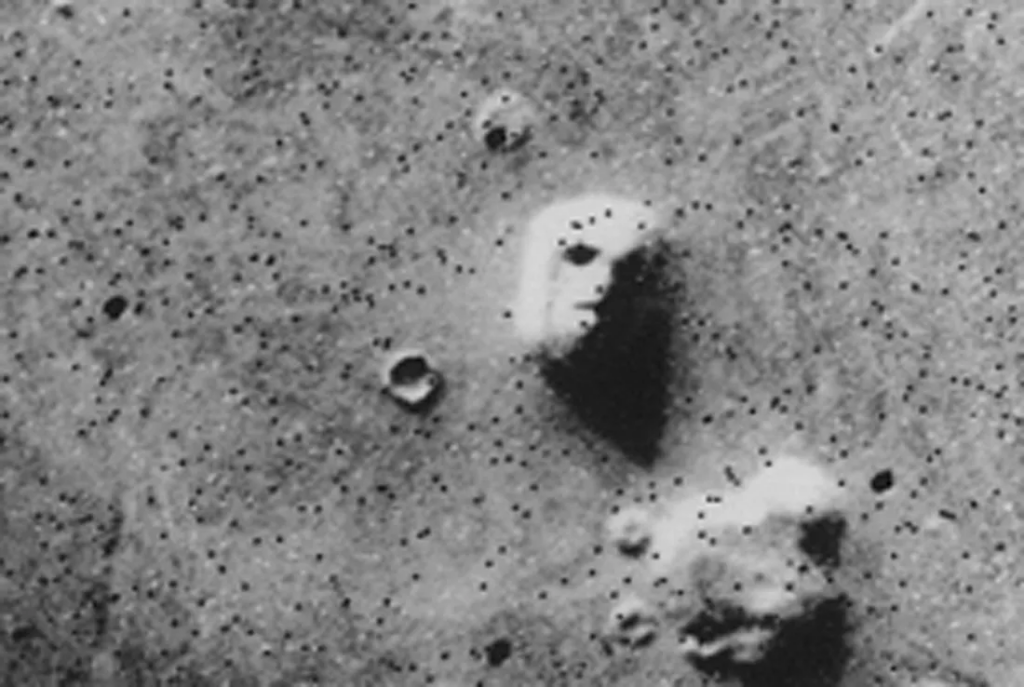
Humans have a tendency called pareidolia, where we perceive familiar patterns—like faces—in random stimuli. Have you ever seen a face in the clouds or the man on the moon? A 2009 study about pareidolia explored how our brains are wired to recognize faces, sometimes leading us to “see” them where they don’t exist. This is comparable to how LLMs might generate meaningful-sounding text that isn’t based on factual data.
Neurological phenomena like phantom limb sensation demonstrate the brain’s ability to create vivid experiences without external stimuli. Individuals who have lost a limb often feel sensations, including pain, where the limb used to be. Similarly, déjà vu gives us the illusion of familiarity in entirely new situations. These experiences underscore the brain’s role in constructing reality, much like how LLMs generate content based on learned patterns rather than grounded input.
Want to read more on pareidolia between humans and computers? Pareidolia: A Bizarre Bug of the Human Mind Emerges in Computers – The Atlantic
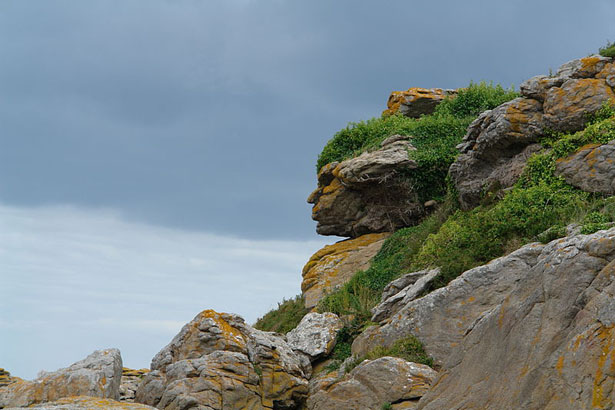
Conclusion
Recognizing that humans are more like LLMs than we might like to admit opens the door to a deeper understanding of both artificial intelligence and ourselves. The similarities in how we process information, fill in gaps, and sometimes generate inaccuracies highlight fundamental aspects of intelligent systems—be they carbon- or silicon-based. By embracing these parallels, we can refine AI technologies and become more mindful of our own cognitive processes, ultimately leading to a more nuanced appreciation of intelligence in all its forms.
Leave a Reply